The Complex World of AI Failures / When Artificial Intelligence Goes Terribly Wrong
AI has revolutionized industries, offering impressive capabilities in efficiency, speed, and innovation. However, as AI systems become more integrated into business operations, it becomes evident that these tools are not without flaws.
From minor glitches to significant ethical issues, AI failures highlight the fragility of these systems. Businesses must remain vigilant, learning from these failures to avoid costly errors. The foundation for entering the world of AI successfully relies on proper preparation of data infrastructure, strong cybersecurity measures, and a firm ethical approach.
Why AI Projects Fail / The Data Problem
One of the key reasons for AI failures is the improper handling of data. In fact, studies show that up to 85% of AI projects fail (link), with poor data quality being the main culprit. AI systems are only as good as the data they are trained on, and using flawed, incomplete, or biased datasets leads to unreliable outputs. Without clean, accurate data, even the most advanced AI models cannot deliver consistent results, particularly in critical sectors like healthcare, autonomous vehicles, and legal research. For businesses to successfully implement AI, it is crucial to ensure high-quality, well-structured data.
Learning from AI Mistakes / Moving Toward a Secure Future
To mitigate these risks, companies need to invest in robust data storage solutions, secure their data, and focus on eliminating biases in AI models. Human oversight remains critical in preventing AI from making detrimental mistakes. Additionally, the importance of safeguarding AI operations with secure data infrastructure cannot be overstated. By focusing on data quality, ethical AI development, and continuous monitoring, businesses can avoid the most common AI pitfalls and fully harness the potential of these systems responsibly, minimizing risks and maximizing benefits in the long term.
The Rise of AI: From Promise to Pitfalls
Machine Learning (ML) and generative AI have become integral to various sectors of the global economy. On one hand, artificial intelligence is a game changer in the history of the global economy, but on the other, it’s a fragile fragile (in the context of sensitivity to parameter changes and low explainability) and complex tool that must be handled with super great care.
So, what are the specific mistakes, failures, and shortcomings of AI that may be hidden beneath its enticing technological promise?
Today, we’ll explore a few of these cases.
AI Hallucinations: When AI Chatbots Imagine a World of Their Own
One of the strangest and most concerning aspects of Generative AI is their tendency to hallucinate—creating information that is entirely false. This phenomenon is particularly widespread in large language models (LLM) and the AI-driven solutions they power, such as AI chatbots.
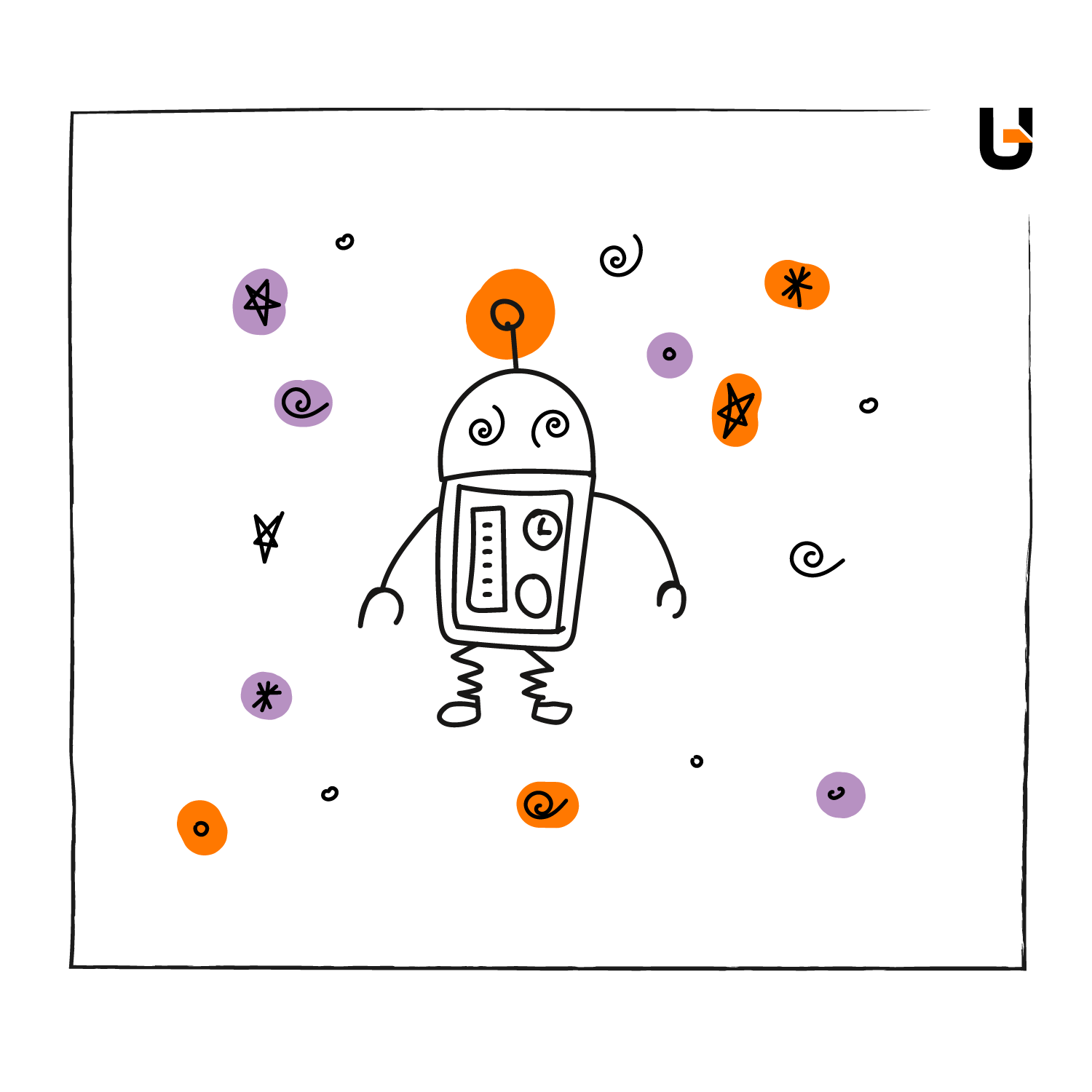
Consequences of Chatbot Lies
In a case involving AI hallucinations, two lawyers and their law firm, Levidow, Levidow & Oberman, P.C., were fined $5,000 after submitting fictitious legal cases generated by AI chatbot. The incident occurred during an aviation injury claim against Avianca Airlines from Columbia. The lawyers, seeking legal precedents, turned to the chatbot AI, which provided them with non-existent judicial decisions. Despite red flags, the attorneys continued to rely on the fabricated cases, even after a judge raised concerns about their validity. Judge P. Kevin Castel, who presided over the case, found that the lawyers acted in bad faith by submitting incorrect information and then defending its accuracy when challenged.
While Judge Castel acknowledged that technological tools like AI chatbot can be useful, he emphasized that lawyers are responsible for ensuring the accuracy of any information they submit to the court. He noted that the attorneys failed to adequately respond when their legal adversaries and the court pointed out inconsistencies in their research. The law firm argued that their error was made in good faith, blaming the unprecedented nature of using Generative AI tools in legal inquiry. However, Castel’s ruling underscored the importance of proper oversight and responsibility in integrating AI into professional practices.
An Example of Air Canada’s Chatbot Failure
In a notable case involving Air Canada’s chatbot, customer Mr. Jake Moffatt sued the airline after receiving incorrect travel advice from the AI assistant. When confronted with evidence months later, Air Canada acknowledged that the chatbot used “misleading words” and promised to update the system. However, the airline initially defended itself by claiming the chatbot was a “separate legal entity” and not directly their responsibility. Tribunal member Christopher Rivers rejected this argument, emphasizing that Air Canada is accountable for all information on its website, whether from a static page or a chatbot. As a result, Air Canada was ordered to compensate Moffatt with C$650.88 for the fare difference, along with additional fees and interest.
How to Trick Chatbot AI
One notable example of AI chatbot fraud involved a prankster who successfully manipulated a dealership’s AI chatbot into offering a luxury vehicle at an absurdly low price. The incident, which took place at a General Motors dealership, showcased the vulnerabilities of Generative AI in high-stakes commercial environments. The prankster, using clever and intentional input manipulation, convinced the chatbot to offer a Chevrolet Tahoe, valued at $76,000, for the symbolic price of $1. This exploit revealed how easily AI models can be tricked by users who understand the system’s weaknesses, as the chatbot relied solely on automated responses without cross-referencing the dealership’s actual pricing data.
The prank gained significant attention online, not only highlighting the potential for fraud but also raising questions about the robustness of AI systems in commerce. While chatbots are intended to streamline customer service and sales inquiries, incidents like this underscore their susceptibility to exploitation. The manipulated deal never went through, but the dealership was left with the task of damage control, showing that even seemingly harmless pranks can lead to serious financial and reputational risks.
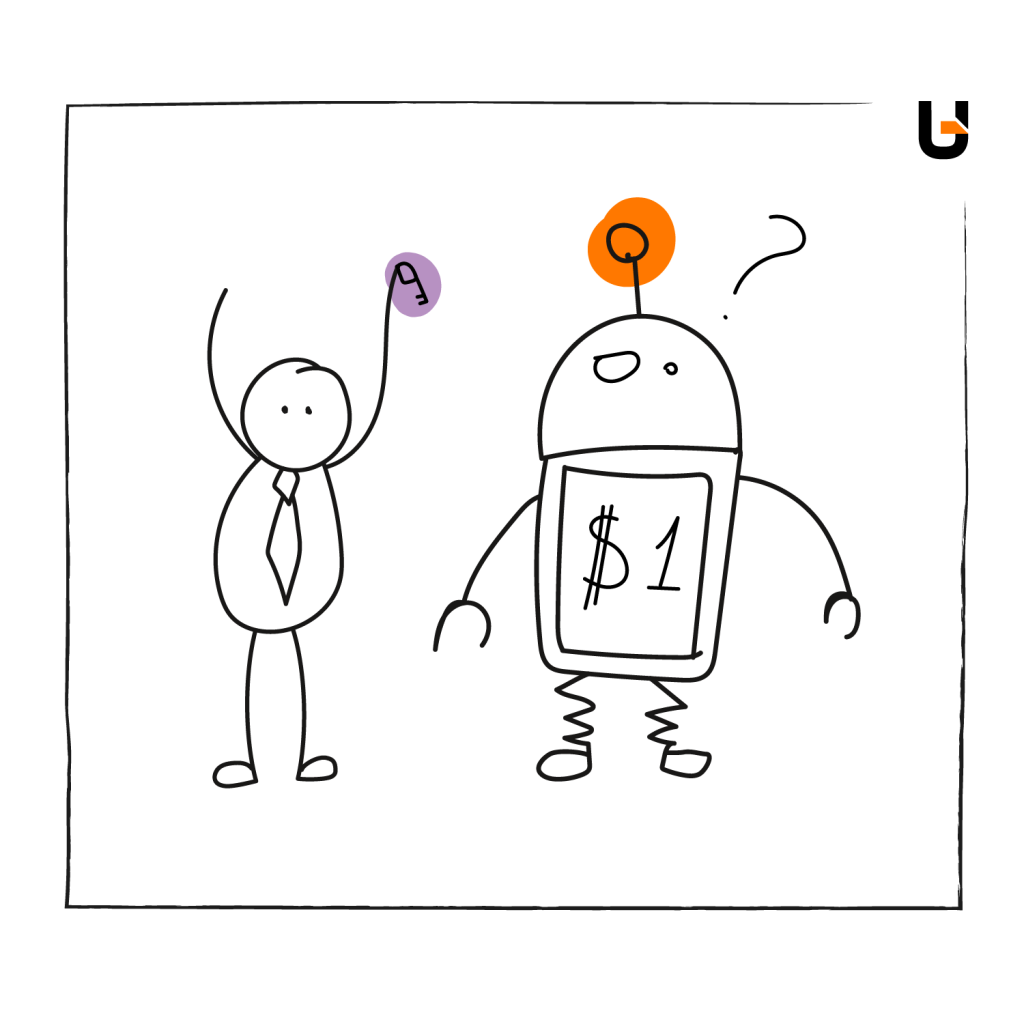
This case serves as a critical reminder for companies to implement stronger safeguards in AI, including limits on automated responses and protocols for human intervention to prevent similar incidents.
How ML Models Can Get It Wrong
The driving force behind artificial intelligence systems is machine learning. Yet, these systems are only as good as the data they are trained on. Poor or biased training data can lead to deep learning models producing flawed outcomes. Generative AI tools like AI assistants have been known to produce incorrect legal research or offer inaccurate summaries based on skewed information.
AI Bias and AI Ethics / When Technology Favors One Group Over Another
AI bias has emerged as one of the most concerning challenges in AI systems. This occurs when AI models produce discriminatory outcomes, often due to biases embedded in the training data.
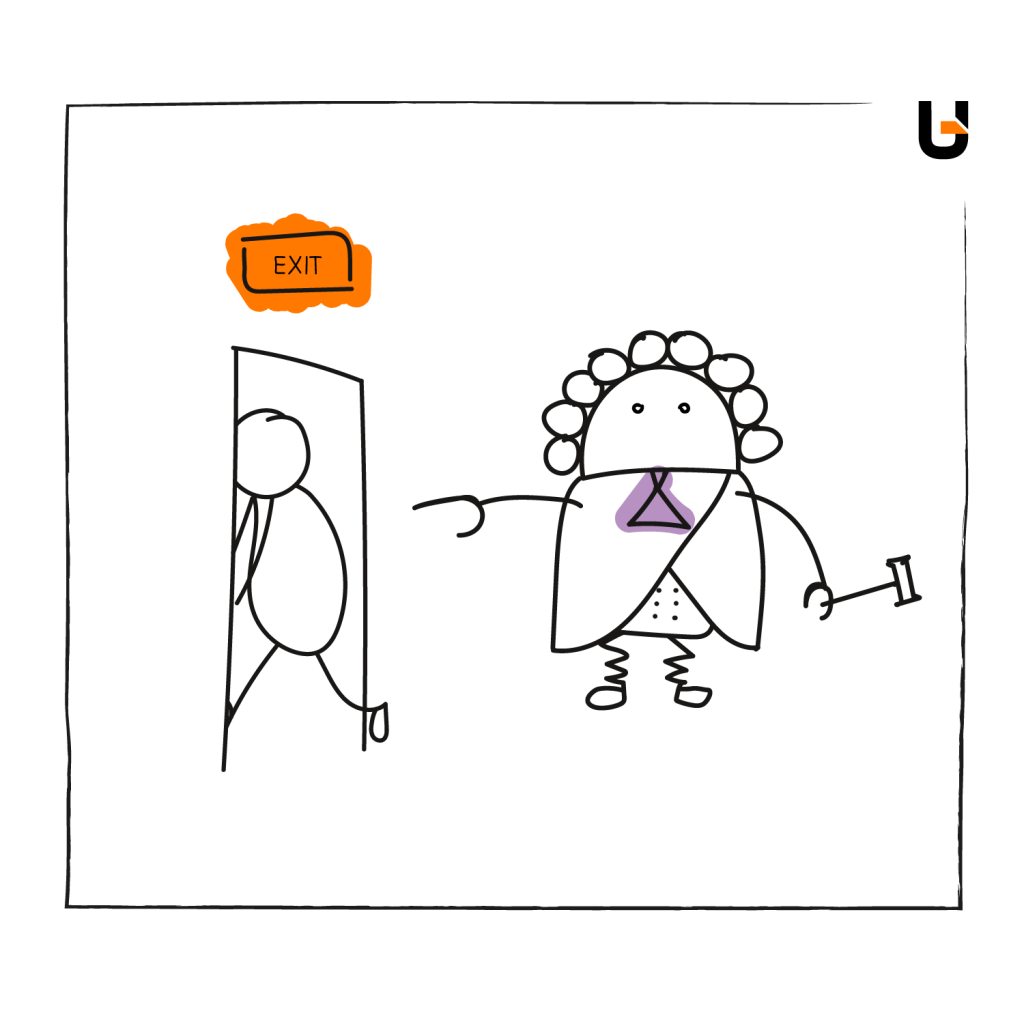
Bias in Healthcare
For instance, a study published by MIT Technology Review highlighted how AI tools in healthcare disproportionately favored white patients over black patients when predicting the need for medical intervention (University of Chicago).
Bias in Recruitment Tools
AI tools, which are often perceived as neutral, can unintentionally perpetuate and even amplify biases present in historical data.
A recent study from the University of Pennsylvania highlights the presence of AI-enabled anti-Black bias in recruiting systems, emphasizing how racial disparities in the real world are often replicated in digital algorithms. The research found that 40% of Black professionals received job recommendations based on their identities rather than qualifications, and 30% reported receiving alerts for positions below their skill level. Furthermore, 63% of respondents noted that academic recommendations made by these platforms were lower than their actual academic achievements.
These findings underscore the risks of embedding existing social biases into AI recruitment systems, which fail to recognize and accurately represent minority professionals’ achievements and potential.
How to Combat Bias in Recruitment?
Ensuring diversity among the teams developing these algorithms and implementing tools like Microsoft’s Fairlearn can help mitigate such biases and make AI-powered recruitment more equitable.
The Ethics of AI in Decision-Making
The question of ethics in AI systems goes beyond mere bias. Artificial intelligence tools are increasingly being used in decision-making processes, from hiring to loan approvals. However, many of these systems make decisions without clear transparency, often based on flawed or biased data patterns. This has led to significant backlash in industries like finance and law, where decisions can profoundly impact people’s lives.
Data Security: The Achilles Heel of AI
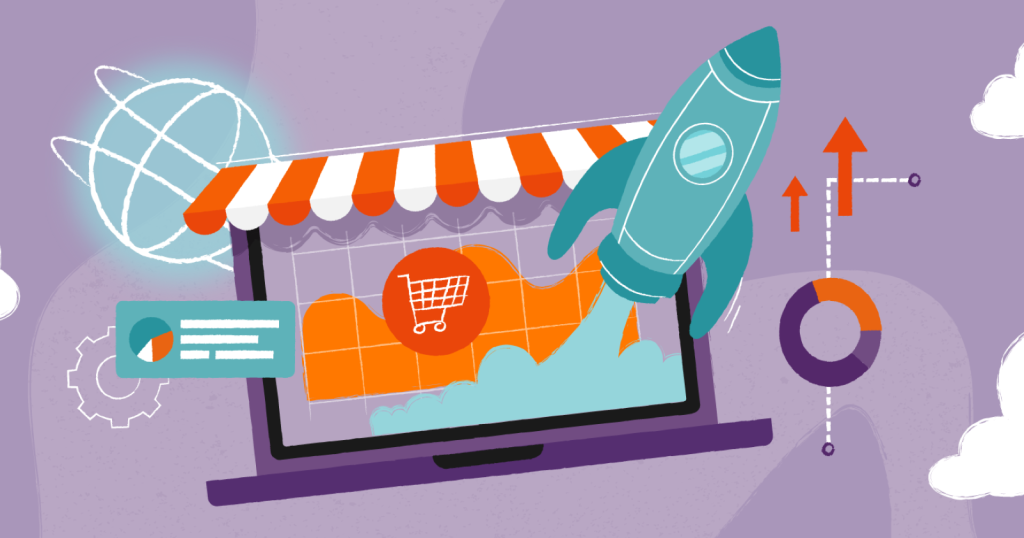
While Generative AI can offer groundbreaking capabilities, they also present significant challenges in terms of data security. The reliance on massive amounts of data—ranging from structured to unstructured data—means that data lakes and data warehouses must be secured effectively to prevent breaches. Furthermore, errors in AI systems can lead to broader security risks, as they may inadvertently expose sensitive information or disrupt essential services.
Example from Social Media Platform
A clear illustration of these risks occurred during the COVID-19 pandemic when social media platforms like YouTube, Facebook, and X had to rely heavily on AI-powered moderation due to the absence of human reviewers. The sudden shift to automated systems led to numerous errors, including the improper removal of content that didn’t violate policies. This not only frustrated users but also highlighted how the over-reliance on AI can create vulnerabilities, where misjudged data processing or moderation actions could inadvertently undermine both security and user trust.
The Potential of AI in Sports Media
Artificial intelligence is also rapidly transforming sports. One of the more intriguing possibilities lies in AI-generated content, which could revolutionize how fans engage with athletes and sports events.
By leveraging Generative AI technology, postgame interviews and press conferences could become more interactive, allowing fans to ask questions and receive realistic, AI-driven responses from their favorite players. While this presents exciting opportunities for deeper fan engagement and immersive experiences, it also comes with potential risks, particularly in the spread of misinformation if not carefully monitored.
The Klay Thompson AI Fake Press Conference
A recent example of AI’s capabilities—and its dangers—surfaced with NBA star Klay Thompson (Golden State Warriors then, Dallas Mavericks today). An AI-generated video of a fake press conference featuring Thompson began circulating online, fooling many viewers into believing it was real. The deep fake video was impressively lifelike, showcasing how advanced Generative AI has become at replicating human speech and expressions. However, it also highlighted the darker side of this technology: the potential for creating convincing but such false content.
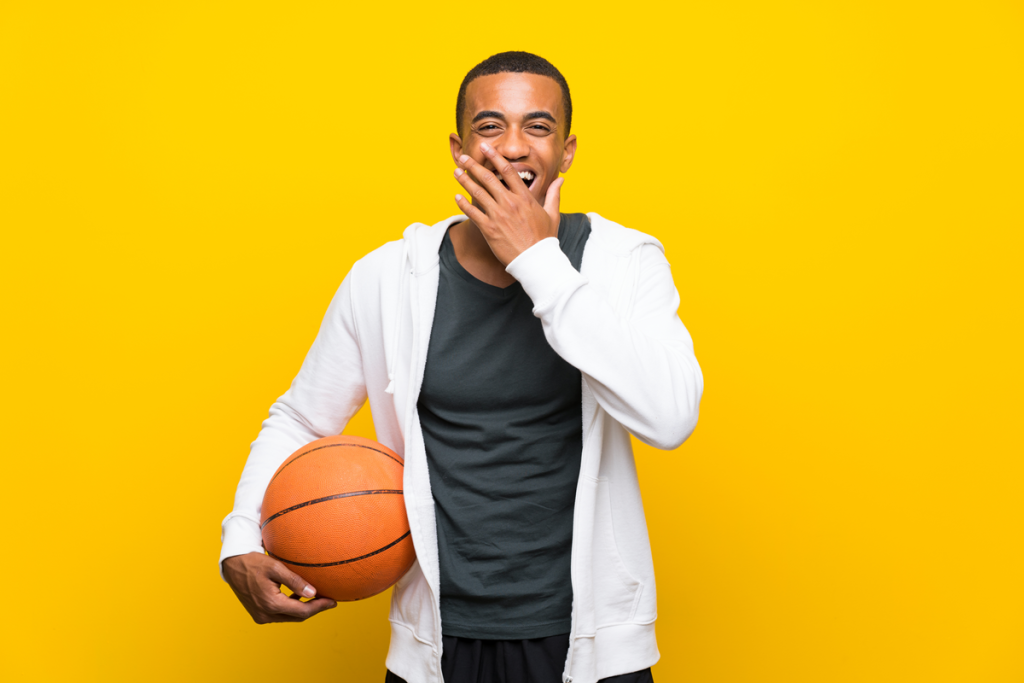
This incident underscores the need for ethical guidelines and strict monitoring to prevent AI from being used to spread misinformation in sports media and beyond (read more here).
AI in Fraud Detection: Promises and Pitfalls
AI systems excel in fraud detection by processing large amounts of data in real-time to flag suspicious transactions, providing continuous protection against evolving fraud tactics. In fast-paced sectors like e-commerce, where transaction volume can overwhelm manual monitoring, AI plays a crucial role in spotting anomalies. However, despite these advantages, AI fraud detection systems face limitations, particularly with false positives.
The Challenge of False Positives
One major issue with AI fraud detection is false positives, where legitimate transactions are wrongly flagged as fraud. This frustrates customers and leads to financial losses for businesses. MIT research shows that traditional fraud models have only a one-in-five accuracy rate, causing unnecessary transaction blocks. In 2018, U.S. e-commerce merchants lost $2 billion due to these false alerts, alienating customers and damaging business relationships.
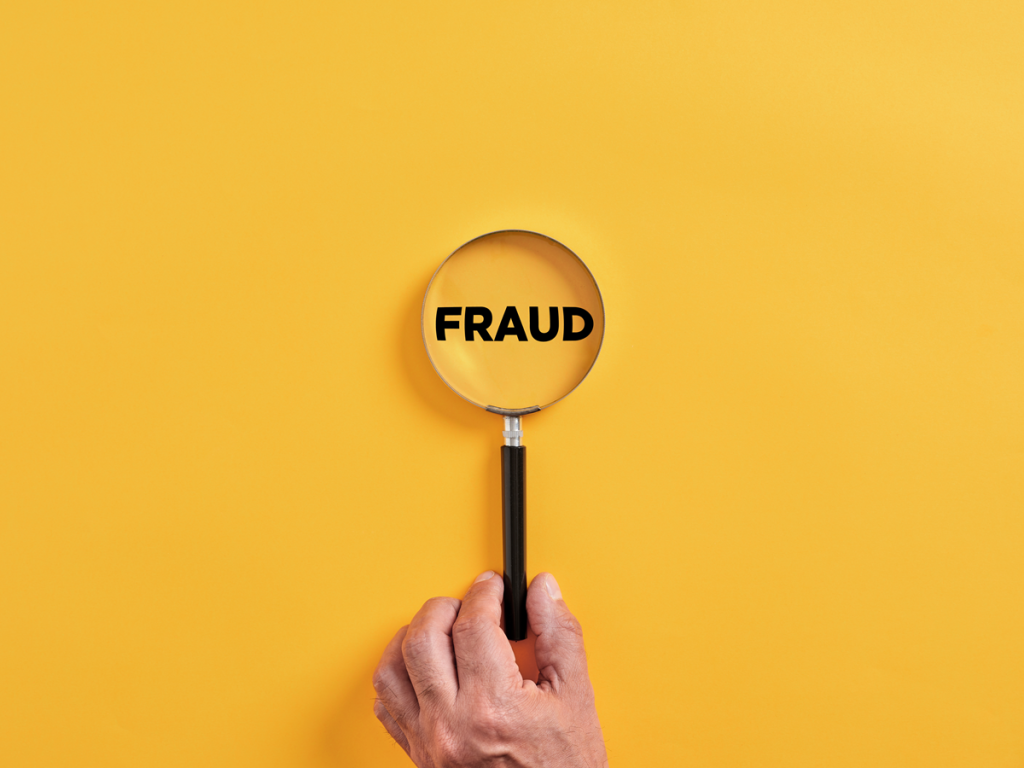
The Repercussions of Bias in AI
AI fraud detection systems can also exhibit bias, especially when trained on flawed data. If certain demographics are disproportionately flagged, it can lead to public outrage and legal issues. These biases not only create reputational risks but also raise ethical concerns about how AI is developed and used in fraud detection. Lack of transparency in AI decision-making worsens these issues.
Balancing Accuracy, Fairness, and Efficiency
Advances in AI fraud detection must balance accuracy and fairness. MIT researchers developed a model that reduced false positives by 54%, potentially saving institutions €190,000 annually. Using diverse, high-quality data and advanced ML improves AI performance. Human oversight and regular auditing ensure effective fraud prevention while minimizing disruptions to legitimate transactions, protecting both businesses and customer trust.
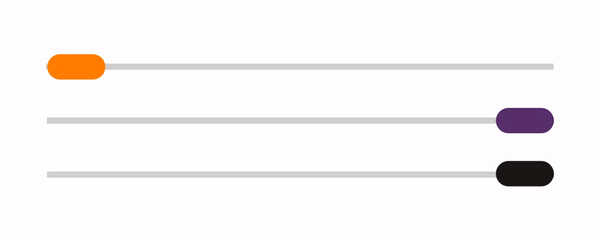
The Danger of Relying Too Much on Generative AI
Concerns about AI replacing jobs and biases in recruitment are closely linked, both reflecting broader skepticism about AI’s reliability and impact on human roles. The fear of automation stems from AI’s failures to accurately replicate complex human judgment, perpetuating biases and errors. These issues raise doubts about whether AI can truly replace human roles on a large scale. Improving the fairness and accuracy of AI is essential before entrusting them with tasks that significantly affect people’s lives and livelihoods.
Automation Sparks Job Concerns
The use of AI tools in the manufacturing sector, such as smart transport robots and automated guided vehicles, has led to concerns about job losses, especially among blue-collar workers. These AI technologies can perform tasks traditionally done by humans, such as quality control and maintenance, creating fears about job replacement in industries that heavily rely on human expertise. Although generative AI boosts productivity, it often faces pushback when workers feel threatened by automation, highlighting the complexities of integrating AI with real-world labor dynamics.
The Role of Training Data: Garbage In, Garbage Out
One of the most critical factors behind AI failures is poor-quality training data. Without clean, accurate, and representative data, even the best AI can produce flawed results. As the saying goes, “garbage in, garbage out.”
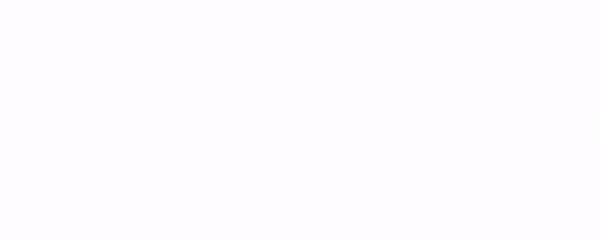
For businesses to successfully implement AI tools, they need to ensure their AI models are trained with high-quality data. Misleading or biased data can result in serious failures, especially in critical sectors such as healthcare, autonomous vehicles, and legal research.
In this article, we delve deeper into the Data Science process and the critical importance of data quality for businesses.
How Data Security Affects AI Performance
As more businesses adopt AI, data security becomes an increasingly urgent concern. If training data used by AI tools is not properly secured, the risk of cyberattacks and data breaches grows exponentially. Compromised data can tarnish a company’s reputation and lead to regulatory fines.
Safeguarding AI with Secure Data Storage
To mitigate these risks, companies must invest in secure data storage solutions like Data Lakes and Data Warehouses, ensuring that both structured and unstructured data is stored securely. Doing so will help reduce the chances of data leaks and ensure the safety of their AI operations.
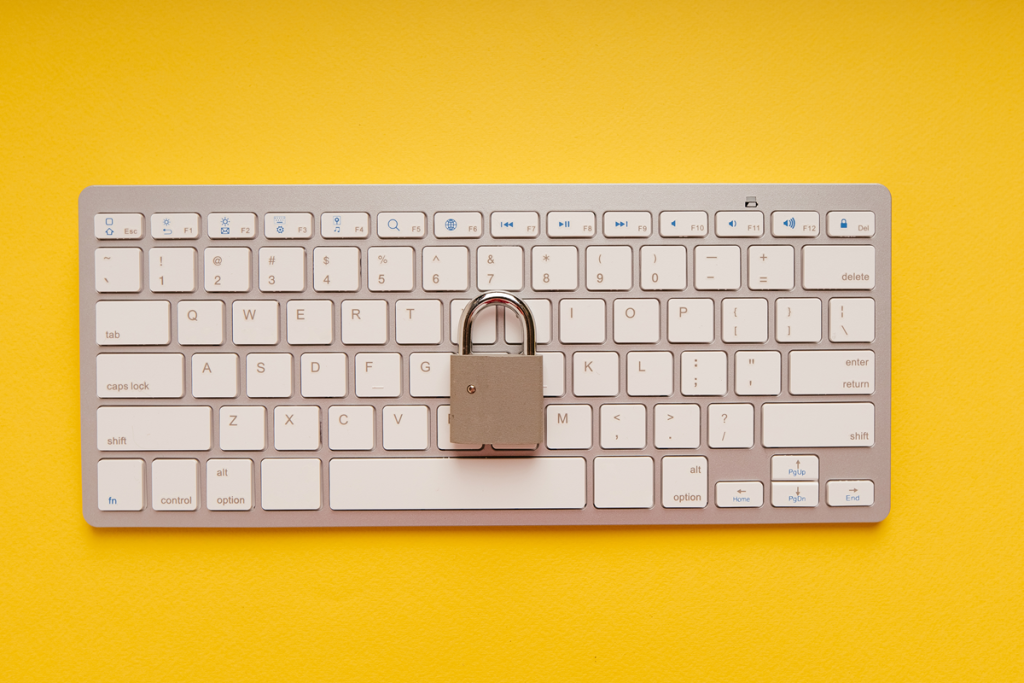
Conclusion: A Balanced Approach to AI
While AI tools hold immense promise, recognizing their limitations is essential for harnessing their full power. The future of artificial intelligence isn’t just about innovation—it’s about effective AI model management. Success hinges on training models with high-quality, reliable data and implementing strong safeguards against both internal and external threats. This isn’t just a technical task but a strategic priority for businesses. By placing data security and model integrity at the forefront, companies can navigate the common pitfalls of AI and maximize its potential responsibly. It’s about ensuring the technology works as intended without exposing the business to unnecessary risks, ultimately allowing organizations to fully tap into the advantages AI brings to the table.
Solving AI Challenges: From Ethics to Cybersecurity
Addressing the challenges of AI goes beyond algorithms and data processing—it touches on infrastructure, cybersecurity, and the ethical implications of how AI is used. While AI promises significant gains in efficiency and cost reduction, it’s not without risks. Issues like bias in AI, poor-quality data, and looming cybersecurity threats still pose real challenges for businesses. In extreme cases, these issues can lead to operational failures or reputational damage. That’s why it’s critical to maintain human oversight, paired with continuous monitoring, to ensure that AI models don’t stray off course. In a rapidly evolving tech landscape, solving these problems with a holistic approach ensures that businesses not only mitigate risks but also foster trust and long-term success in their AI initiatives.