Data Science for Retail / A Guide with Use Cases
Imagine a mid-sized supermarket in a world without customer analytics tools. Every customer who enters the store remains a mystery. His or her expectations, interests, needs, and past purchases are unknown. The owner must rely on intuition and their gut feeling to guess which products to place on the shelves, which promotions to run, and how to serve the customer.
Fortunately, today there is no need to guess. Instead, you can rely on reliable data and advanced tools. This is all made possible by customer analytics with advanced data science and machine learning solutions.
What is Data Science in Retail?
Data science in retail involves the tracking of customer behavior based on demographics, historical transaction data, customer interactions and more. Based on this data, it is possible to understand customer behaviors, create general behavior patterns for specific groups of people and generate actionable insights.
Such data analyses in retail business allow you to:
- Identify customer expectations and apply a personalized approach to each of them
- Create a more effective marketing and sales strategy for current and prospective customers
- Effectively apply precise customer segmentation, resulting in more targeted marketing activities
- Improve sales and prevent customer churn
- Anticipate and respond to customer needs
- Deliver personalized offers to improve sales metrics, including average order values (AOV) and customer lifetime values (LTV).
How to Get Started with Data Science in Retail
Before we show you practical examples of how to apply data science tools to the retail industry, let’s pause for a moment! To get started with data science techniques, there are a few things you need to keep in mind.

1 / Data
The foundation for advanced customer analytics is data and the tools to collect it.
Your customer data can come from a variety of sources: CRM system, sales system, website analytics, social media, market research, loyalty cards and so on.
It’s a good solution to create a single source of truth so that the data is always up-to-date and in one place. For large amounts of data, consider using a data lake or data warehouse. These tools make it easier for analysts and data scientists, among others, to access this data, quickly explore relationships between various datapoints, and draw meaningful conclusions.
2 / Business Intelligence Tools
To analyze data from multiple sources, it is a good idea to implement data analysis platforms (Business Intelligence tools) that allow you to quickly and effectively analyze and visualize larger data sets. BI platforms are commonly used to create interactive dashboards and reports that allow you to create interactive dashboards and reports that provide useful insights.
One of the most popular tools is Power BI, which lets you integrate multiple data sources-files, databases, online services, and more. With Power BI, you can effectively visualize and report on any data in your organization.
3 / Data Science Process
If you already have data collection tools in place and are already analyzing metrics relevant to your business, you can move to the practical implementation of data science in retail.
The key here is to identify business needs, understand and prepare data for the algorithm. The data scientist will then build a model to test against your business assumptions, starting with a smaller sample of data.
Remember that the data science process has “science” in its name for a reason. It is an iterative process of hypothesis testing that should ultimately lead to tangible business benefits.
Data Science Tools in Retail Industry
Let’s now take a look at specific data science tools used in the retail industry and see how these solutions can be practically applied to both B2C and B2B business. We will approach the topic from the perspective of challenges and present data science applications.
Challenge 1 / Untargeted, Haphazard Marketing That Burns Budgets and Fails to Deliver Results
Solution: Loyalty Card Profiling
With loyalty cards, sales and marketing can learn more about a customer’s buying habits and assign them to a specific segment. This knowledge allows for more personalized marketing activities.
The method is based on stratification, which is the assignment of customer groups to independent sets. Such an assignment is made using a clustering algorithm. Customers assigned to a particular cluster have their own specific characteristics, which makes it possible to create ideal offers for the right segments. Customer segments can form the basis for a product recommendation system or marketing content.
Challenge 2 / High Percentage of Customer Churn
Solution: Survival Analysis
It’s not uncommon for the retail industry to have problems with customer churn and not know when – and which – customers are likely to leave. But what if it were possible to know in advance when certain customers are most likely to part ways? The company could respond with an appropriate offer, discount, new contract terms, etc. After all, it is more expensive to acquire new customers than to retain existing ones.
Survival analysis is based on analyzing the average length of stay in a loyalty program. In business, such an analysis can be used to determine the probability associated with how long a customer will use your services and what factors influence this.
By analyzing a customer’s historical data and purchase decisions, you can predict churn. As you analyze offline and online transactions, you may find that customers whose average purchase per month (or other period) falls below a certain threshold are more likely to churn.
For customer service, marketing, and sales development staff, this is very useful information that allows you to take specific corrective action. It’s very much worth the effort, as increasing customer retention by as little as 5% can result in profit increases ranging from 25% to 95%.
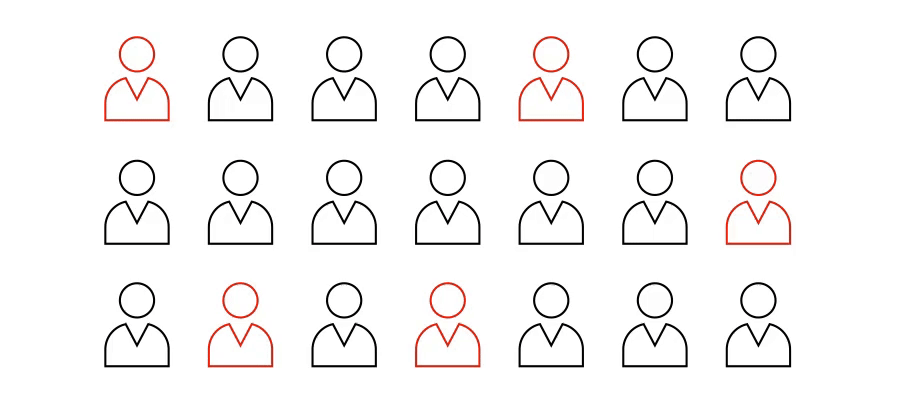
Challenge 3 / Unsatisfactory Shopping Cart Values
Solution: Recommendation Engines
Recommendation engines have been around for more than a dozen years, and they continue to evolve and improve. Customers appreciate personalized offers, as shown by Amazon’s data cited by McKinsey – 35% of purchases are made through relevant recommendations.
In the case of recommendation engines, we can talk about a number of different recommendations, such as product pair recommendations, where the recommendation algorithm suggests the next product to the customer. This method is based on the sales history of individual products. Very often, products are sold to customers in pairs. The algorithm captures such pairs and determines the probability of their recurrence.
This method can be extended with additional personalization based mainly on customer segmentation. According to the previous analysis, a customer who buys product X can be assigned to a certain segment.
For example, a construction company owner who buys plasterboard for walls belongs to a different segment than a roof insulation specialist who also buys plasterboard. Although they both buy the same product, they need different accessories.
Challenge 4 / Low Revenue per B2B Customer
Solution: Create personalized shopping carts and offers in B2B.
Again, we turn to the recommendation engine and the use of mechanisms to identify products that a customer is likely to buy in the future. This can be done by studying the customer’s interactions and historical sales data. Such data analyses will allow you to prepare a personalized shopping cart on the site or an offer that meets the customer’s needs.
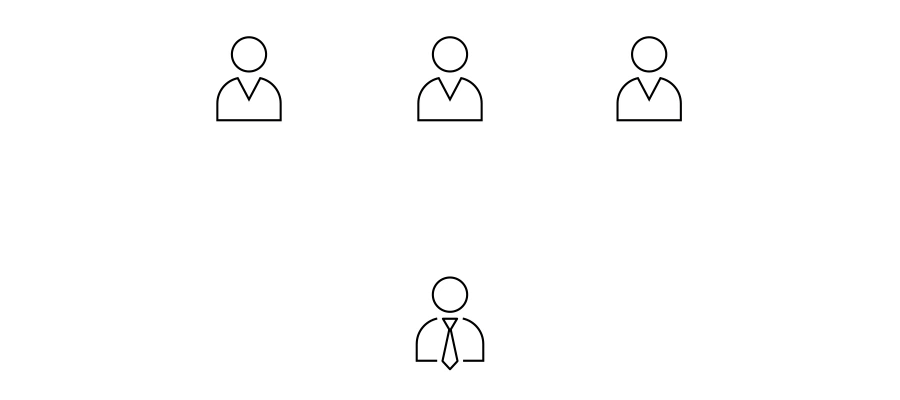
Challenge 5 / Low Sales Efficiency in B2B
Solution: Recommendations on customers for B2B salespeople.
If traditional sales account for a large portion of a company’s revenue, it’s a good idea to suggest to salespeople what actions they can take with certain groups of customers.
With advanced data analytics, reps in the retail sector can learn which products are most important to the customer, which users are less active than usual, or which users are buying only one of a pair of products (and probably the other somewhere else).
Such aggregated information about customers will speed up the work of salespeople and allow them to take more targeted actions based on the data. They can offer the customer (or group of customers) tailored products and take informed action to retain or win back key customers.
With Data Science Tools, the Only Limit is Your Imagination.
Data science in retail is extremely useful for customer needs analysis, segmentation, and tailored advertising. Customers today expect personalization, and data science and machine learning solutions allow you to effectively respond to customer needs.
In this short article, we have described only a small part of the analytical capabilities of data science. We hope that the examples presented here will inspire you to implement new solutions to improve your company’s efficiency, profitability, and sales processes.
Do you want to unleash the power of your data?