Chatbot Analytics / Vital Bot Metrics to Determine Success
Chatbots can do wonderful things. They can enhance the customer experience, save you money, give your business an interactive face 24/7 and more. But ultimately, they have an intended purpose and you need to know how to measure the effectiveness of chatbot solutions in your business.
The term chatbot analytics refers to all of this, naturally, but what exactly do such ‘bot analytics entail? What you want your chatbot to achieve ultimately dictates what metrics to track. So, from the number of users to leads generated, we’ve compiled this list of the most useful chatbot success metrics.
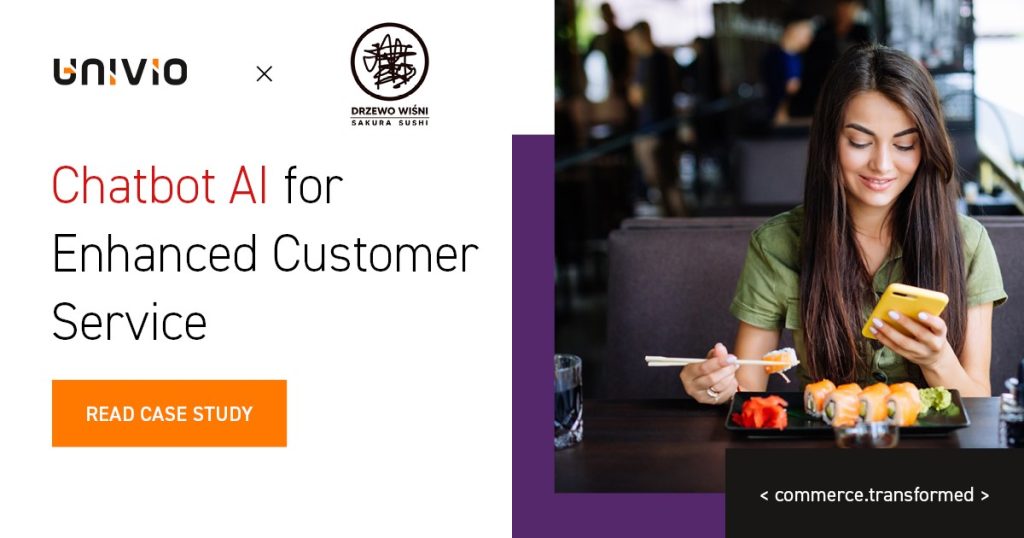
Before we begin: in an ideal world, it’s helpful to know this before you implement your chatbot widget or service. While typical chatbot builders let you update and tweak your chatbot, the core structure should nonetheless be geared towards a goal – and you also need to ensure it accurately measures your chosen metrics.
What Kind of Chatbot Metrics Should You Use?
In our previous blogpost on how chatbots work, we discussed the difference between AI and non-AI chatbots. Many older chatbots are entirely rules-based and based on logic trees or pre-scripted conversation flows.
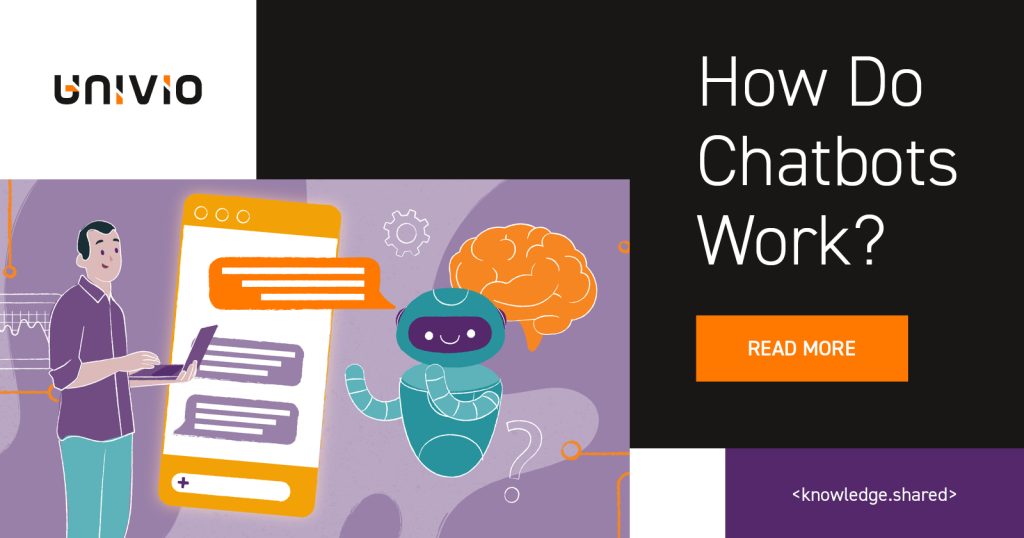
We mention this because the type of chatbot that you implement – as well as the context of your business and the intentions behind your developed chatbot platform – will help determine the right chatbot metrics for your individual case. We’ll highlight, where applicable, where the choice of chatbot influences these performance metrics the most.
It also goes without saying that many of these chatbot evaluation metrics, such as unique users, will likely sound familiar. Chatbots are designed to solve existing problems, similar to an online store or customer service team, so the most important chatbot metrics often overlap with the most common customer satisfaction metrics, too.
The Most Common Bot Metrics to Track
While we’ve listed more than a few metrics, this list is far from exhaustive. We’re focusing on the most important aspects of most chatbot analytics: success rates, customer sentiment analysis and overall performance.
To further help you finetune your chatbot analytics, we’ve separated these metrics into 4 broad categories:
- User Metrics: These initial metrics focus on the general chatbot performance. They show the how often users interact with the chatbot and how it operates as part of your larger business.
- Engagement Metrics: Moving from user metrics, these conversational analytics focus on the level and depth of interaction between your chatbot and its users. These metrics focus on the average performance of your chatbot.
- User Satisfaction Metrics: These metrics track chatbot performance from the perspective of your users. They indicate if your users are satisfied with their interactions, indicating where the customer experience may potentially still be improved.
- Commercial Metrics: As you might have guessed, commercial metrics focus on the chatbots direct impact on business outcomes – specifically financial performance metrics and associated statistics. This includes generating leads and the chatbot’s direct influence on overall revenue.
We’ll follow a similar patten to our previous article on e-commerce metrics to track. Alongside explaining the basics of each metric, we’ll also explore what it can actually tell you and how useful it ultimately is.
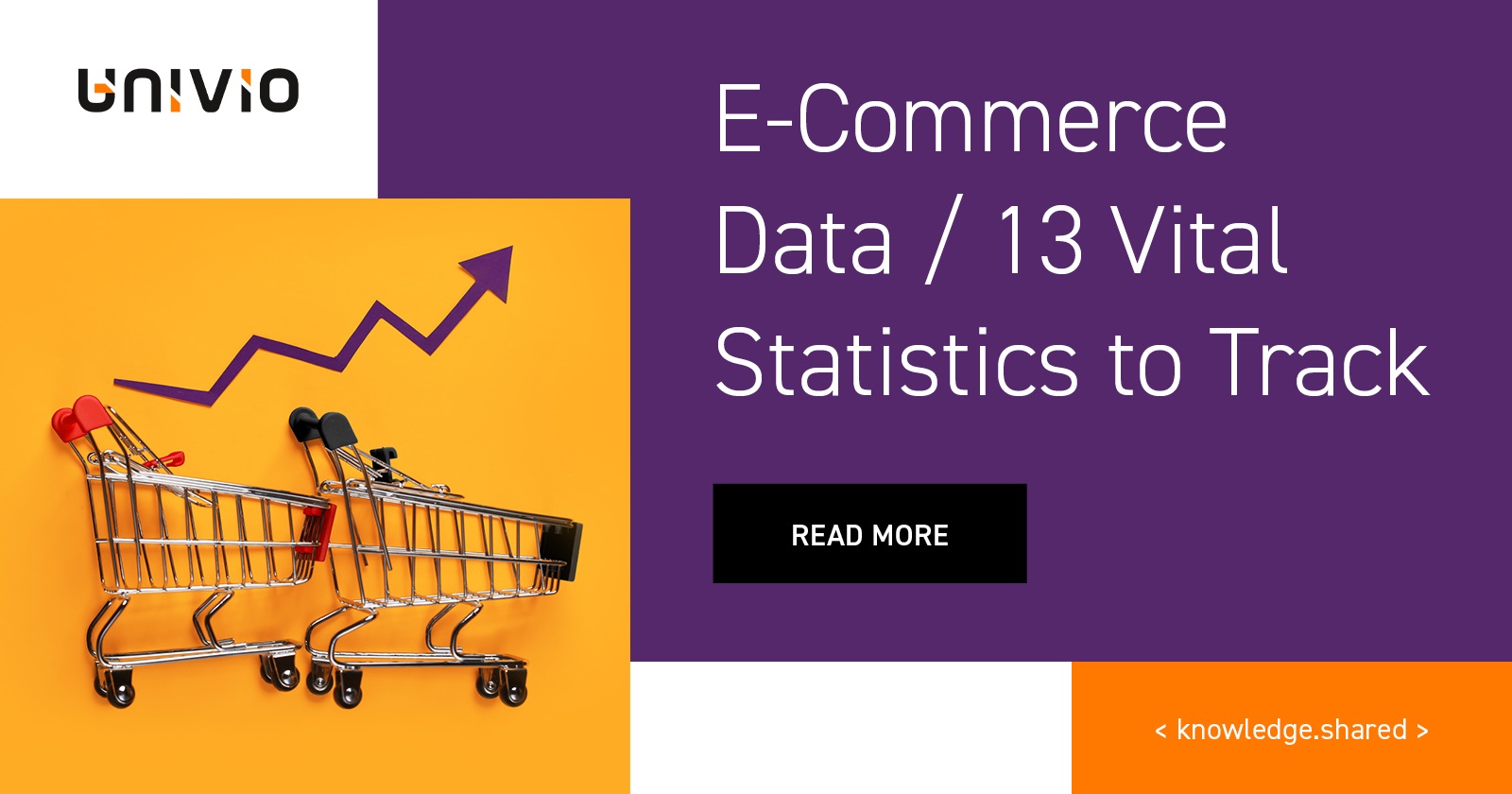
Metrics vs KPIs
While you can have many chatbot evaluation metrics, you should also have a few key performance indicators that tie into your wider business objectives. Not difference is that your chatbot KPIs should have a clear and concise goals – that is, there is a certain level that can be gauged as successful. The wider metrics can show how things are progressive, flag potential errors or improvements to be made, but your most important metrics should also be viewed as KPIs.
User Metrics
The first and most obvious range of metrics focus on user behavior and overall interaction with your solution. These range from vital metrics to good-to-know information on regular business continuity, so there are a lot of worthwhile inclusions for any chatbot analytics tools.
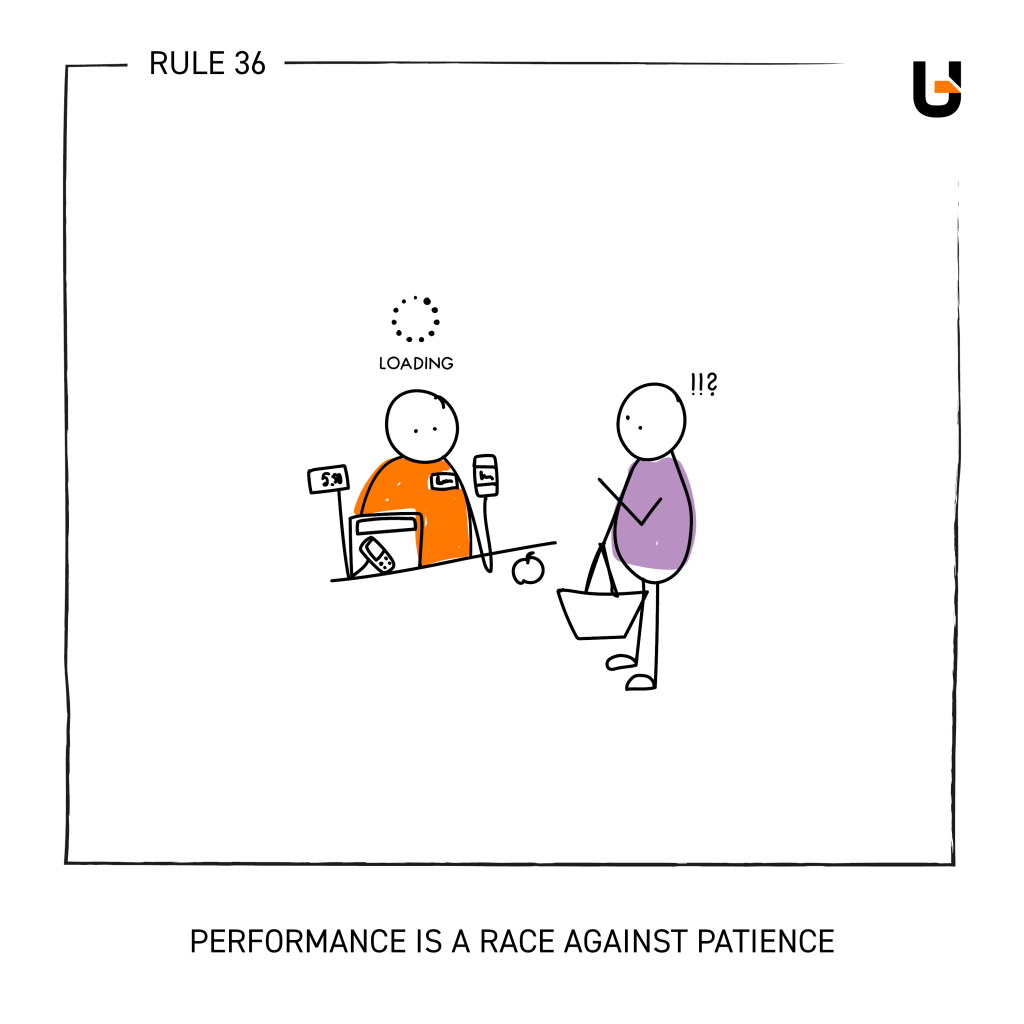
Total Users
Perhaps one of the most obvious metrics in chatbot analytics, Total Users refers to the overall number of users in a given period, be that per day, week, month or even the chatbot’s entire lifetime.
How to calculate it? Most chatbots will count this for you – all you need to do is set the time period that you want to look at. However, thanks to cookieless browsing and the likes of GDPR, you should be aware that Total Users will not be exact. If people who block cookies and other forms of tracking use your chatbot multiple times, then the average number will be higher than reality, since these additional users are essentially fake.
How useful is it? On its own, Total Users doesn’t really tell you much. It can tell you when peak activity occurs and, if your chatbot is present on multiple channels, where most activity takes place. However, when combined with other statistics, Total Users is a very useful metric. It can also aptly serve as a broad measurement of usability over time.
Bots Triggered
This metric tells you how many instances of your chatbot were triggered over a given timeframe. For clarity “triggered” here can be channel-specific. A chatbot on social media is not triggered automatically: the user has to interact with the messenger bot, for example. On the other hand, a chatbot on your landing page typically triggers automatically with a welcome message.
How to calculate it? With most solutions, this metric is automatically measured in the chatbot analytics. Every time the welcome message is prompted, the count goes up. It’s that simple!
How useful is it? Similar to New Users, this is another metric that works well as a comparison tool. For example, even comparing New Users and Bots Triggered on a website-based chatbot can show you how many people utilize the chatbot. There will be some that don’t – they simply see the welcome message and ignore it. This can be worth tracking and potentially something to A/B test.
New Users
Another somewhat obvious metric, New Users tracks users who have not previously used the chatbot. It’s important to note, however, that there is a key distinction between New Users and Unique Users. For example, if you have 1,000 users interacting with your bot, the chatbot analytics could show 100 New Users and 500 Unique Users. The former indicates those who have not previously engaged in chatbot conversations, even outside of the time period, whilst the latter simply refers to known, unique users.
How to calculate it? While this metric sort of takes care of itself, we should stress that it won’t be 100% accurate. A repeat user who uses cookie-less browsing or multiple devices may come across as multiple users.
How useful is it? If your chatbot aims to help users and convert them to customers, then this is a useful metric. It shows how many people turn to the chatbot for the first time in a given period and, thus, the potential for conversions at any given point. For that reason, it’s also useful to compare alongside your general website metrics related to new users as well, to see if there’s any correlation between the two.
Engaged Users
If your business relies on frequent interactions with customers, then this metric is a strong indicator of that success. Knowing the amount of people that come back to the chatbot reflects your overall user retention rate. You may also come across this metric as the “Retention Rate” for that very reason.
How to calculate it? As a metric, Engaged Users depends on your unique business interpretation of engagement. In a business that sells lower value goods on a more frequent basis, engagement could be considered on a weekly basis. On the other hand, for something like a healthcare portal or a business with more infrequent customer activity, such engagement would be much slower. In any case, example criteria to label a user as “engaged” can include:
- A specific number of interactions with the chatbot in a given week or month.
- Average session length – i.e. 5 or more minutes.
- Number of message exchanges per session.
You could also implement criteria based on your business goals, such as completing a purchase. But this can be its own metric for chatbot performance, which we’ll discuss later. It’s also worth noting that the more specific the criteria, the smaller the metric will be. For example, your criteria could include a set session length and a number of exchanges, but requiring both may result in some active users not being considered.
How useful is it? If your business has a solid understanding of customer engagement, this can be an incredibly useful metric. It can determine if your chatbot is replicating the real world situation. For example, in the fashion industry, there may very well be a core demographic that shop with you on a weekly basis, which your chatbot should strive to replicate and support. For such businesses, this can be one of the most critical chatbot analytics measurements.
Active Users
If you’re looking for a metric that shows the overall reach and usage of your chatbot, Active Users is a useful measurement. It shows how many people interact with your chatbot at a very simple level. It shows how many people ask a question, click on a link or otherwise interact at even a singular instance.
It can also be modified for specific time frames, such as Monthly Active Users, which would show how many people make one or more interactions in a given month.
How to calculate it? With a little configuration, it’s easy to track active users – it’s the combination of users that take any action with your chatbot.
How useful is it? On its own, Active Users doesn’t really tell you much, but it’s a good benchmark for deeper insights. It’s useful when compared against Engaged Users, for example. Active Users will show how many people are using the chatbot, whilst Engaged Users will give a better insight into customer satisfaction and long term use. Between Bots Triggered, Total Users, Active Users and Engaged Users, you can already generate a broad overview of the chatbots user funnel and success rates at each stage.
Chat Volume
If you want to know how many messages were sent overall, regardless of specific users or other factors, this is known as Chat Volume. As far as conversational analytics goes, this is quite a raw metric to track, but it does show how many messages are being sent in a given time frame. Note: this includes messages generated by the users.
How to calculate it? Chat Volumes are generated automatically. Every time a user sends a message, or the bot does, then the Chat Volume goes up by one. Of course, this will mean that the end result if somewhat weighted via customer behavior. Users that write in single sentences at a time, rather than paragraphs, will scale your Chat Volume up.
How useful is it? As a simple and broad metric, Chat Volume can be useful for measuring overall productivity in chatbot analytics. For example, your Chat Volume can go up in peak seasons, or even in specific times of the day. This is important to know if you want to improve your chatbot, or ensure its availability and uptime.
Bot Messages
If Chat Volume covers two-way conversational data – i.e. messages from both the chatbot and your users – then Bot Messages refers to only messages sent by your AI bot.
How to calculate it? Like chat volumes, this can be counted automatically, every time your bot sends a message.
How useful is it? Bot Messages are best viewed in regards to the ratio vs User Messages. A high number of bot messages could indicate either detailed responses, or unnecessary, redundant messages. Ultimately it’s very context specific.
User Messages
You’ve probably guessed it already – User Messages refers to messages sent by real people (i.e. users 🙂 ). And, of course, if you combine User Messages with Bot Messages, you get your Chat Volume.
How to calculate it? As with the last two metrics, this can be automatically counted for you. It’s triggered whenever the user sends a message.
How useful is it? Just like bot messages, this is really a sub-metric of Chat Volume. These are a few metrics that only tell you a little, and only in well-defined contexts.
User Sentiment
If you’re using an AI-based chatbot – powered by both Large Language Models and Natural Language Processing (and more specifically, Natural Language Understanding and Natural Language Generation) – then you can track a user’s emotions during chatbot conversations.
How to calculate it? In any given interaction, there will be positive, negative and neutral statements. The NLU within your AI chatbot will measure these. For example, if a customer enters your fashion store and asks why a delivery is late, but adds that they have been a loyal shopper for years, there is obviously a mix of positive (loyalty) and negative (frustration) emotions, as well as neutral statements (such as not receiving a package).
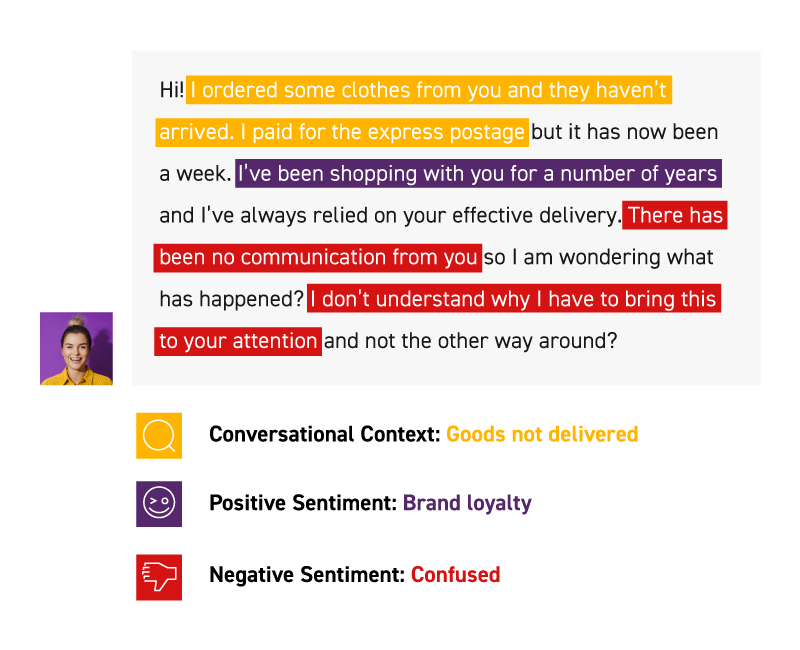
How useful is it? When it comes to chatbot analytics that track customer satisfaction and overall user experience, this is one of the most effective and insightful bot metrics.
The success of this metric, however, depends entirely on the finetuning of your NLU, so it’s worth fully understanding how chatbots work.
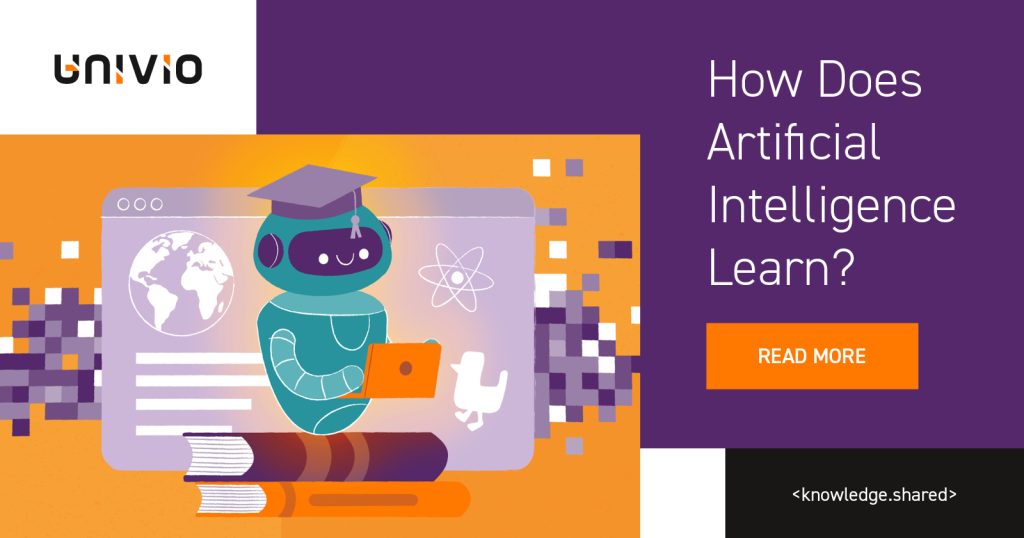
Goal Completion Rate (GCR)
If you’re looking for chatbot success metrics, this does exactly what it says. You set a goal for your chatbot, and then you measure how effective it is.
How to calculate it? An effective Goal Completion Rate needs a clearly defined goal.
- For example, if your chatbot is focused on troubleshooting, then you should consider measuring how many user requests turn into tickets in your backend customer support system. It would not be fair, however, to measure the chatbot via the number of tickets closed – if those tasks go to live agents, you can’t judge the AI by human performance, and vice versa.
- Likewise, if you’re focused on B2C e-commerce, then your chatbot’s goal should be to make sales. The GCR could be expressed by the number of sales, the total amount of revenue generated, or even the revenue per sales. It depends on your wider business strategy.
However, it’s also worth noting that not every objective can be measured entirely within the chatbot. If one of your goals is to save staff time, you would need to measure this through that team’s performance and time monitoring. Chatbot data can show you how much hypothetical time is being saved, but you would need to analyze human performance to know if that improvement is being effectively realized.
How useful is it? We don’t need to tell you that measuring your ability to achieve goals is a key metric. In fact, it’s one of the most important metrics! This can also act as one of your essential Chatbot KPIs.
Engagement Metrics
While the above chatbot analytics provide great insights into general activity, if you want to focus more on granular engagement, there are a few extra conversational analytics that you can look at. These can complement your existing analytics with more insights into the interaction between human users and AI bots.
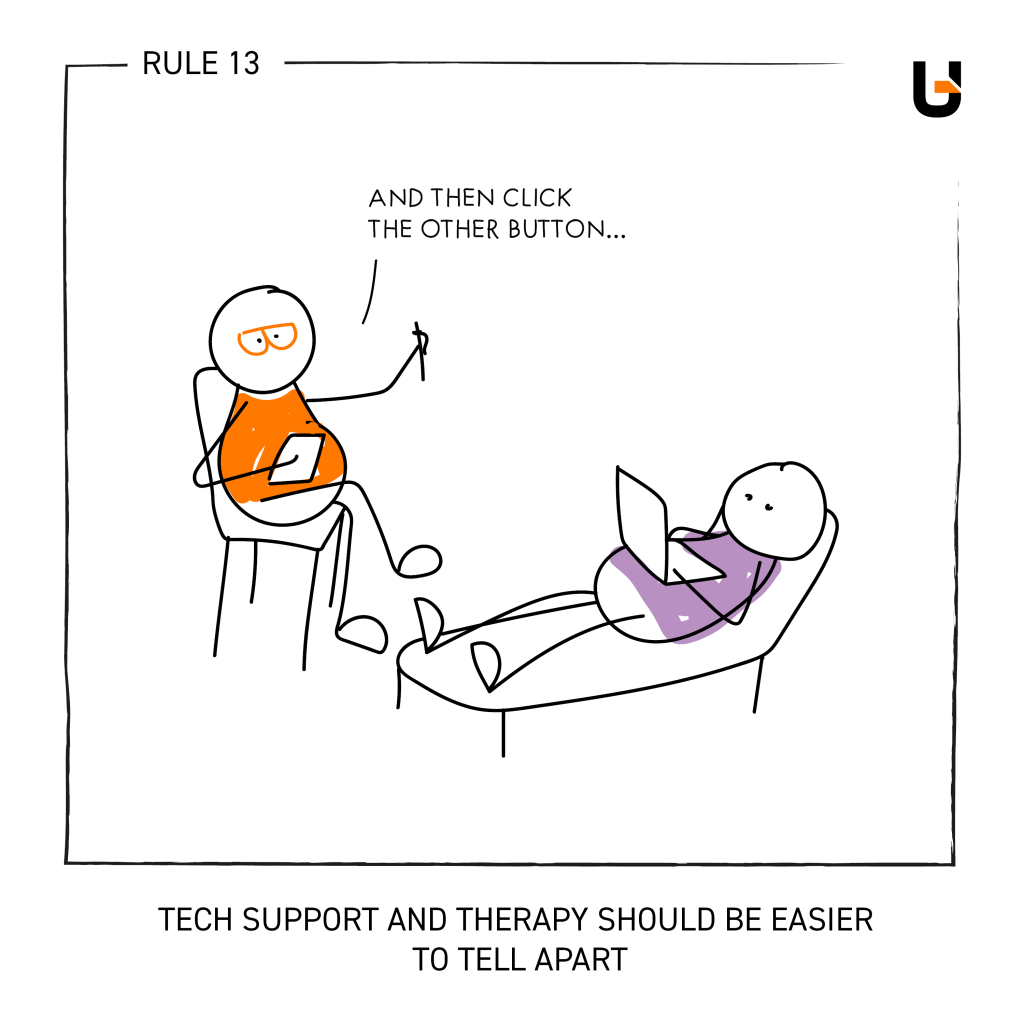
Conversation Length
This is another obvious metric. Conversation Length covers the average conversation length from a given time period. Depending on your chatbot strategy, it can be quite a useful metric.
How to calculate it? Conversation length is generally tracked automatically. It starts when the user first enters a message (this is important if your chatbot sends its own welcome message automatically) and when the user closes the conversation or chatbot.
How useful is it? You can think of conversation length similar to your website’s dwell time, although you should take into account the exact need and purpose of your chatbot. If the bot’s responses are incredibly effective at resolving immediate and clear needs from your target audience, you might find the average conversation length of your total conversations is rather low. In this case, that would not be a bad thing!
In more complex situations, a higher average length could be a sign of more engaged conversations between both the bot and the user. This would need to be compared against your Goal Completion Rate. For example, if your chatbot is based on customer service and troubleshooting, many cases might require a lot of information to solve. You would need to compare your average Conversation Length alongside your GCR to ensure that the final result is still effective.
Bounce Rate
In website analytics, a bounce rate refers to users who leave or otherwise don’t interact with the first page they land on. With chatbot analytics, this metric works very similarly. The bounce rate refers to the number of people that visit the page but don’t engage with the chatbot itself.
Because of this, you can’t use the bounce rate if your chatbot is implemented in the likes of social media, for example. The bot isn’t trigged until someone talks to it, so there isn’t a bounce rate.
How to calculate it? For chatbot analytics, your bounce rate should be specifically measured through strict criteria. This can be measuring users that don’t interact with the chatbot (compared against all users) for example, or comparing all users that message the chatbot against all users that at least open the chatbot widget (if it does not auto-open).
How useful is it? There are many reasons behind a bounce rate, so it can indicate areas for improvement, but be somewhat shy on specific insights. However, this can be adjusted to suit your needs. You might, for example, want to focus more on your website’s bounce rate with chatbot interactions (to see if the chatbot encourages people to stay on the page longer), or focus on bounce rates after 1 message with the chatbot.
Flow Completion Rate (FCR)
In chatbot analytics, the Flow Completion Rate refers to the percentage of users who have successfully completed a predefined sequence of interactions or tasks – the ‘conversation flow’ – with the chatbot. In other words, it measures if the conversation flows are moving along as intended.
How to calculate it? For the FCR to be calculated, you need to track the key steps within your intended conversation flows. When the user completes all of these steps, the flow is complete. Then, by comparing the users that finished the flow against all that at least started, you get your FCR.
Note, however, that a chatbot can have multiple FCRs, as you can have multiple uses for a chatbot. The bot on your fashion store, for example, can help users make a purchase, arrange a refund or enquire about late deliveries. Each of these would be their own conversation flows and FCRs. This is also why we don’t compare against all users – just against the users that triggered each respective flow.
How useful is it? The FCR is most effective when you have predetermined flows and user behavior can somewhat be expected and tailored to. It’s most useful when you have multiple flows, as this can distinguish it from your GCR.
More specifically, if you’re using a rules-based chatbot rather than a more modern AI bot, the FCR is essential for ensuring your intended flows are correct. A low FCR could suggest your flow isn’t working, doesn’t meet user expectations or some other area of improvement.
User Satisfaction Metrics
Next, we have a couple of chatbot metrics to determine how your bot may be perceived by users. Alongside objective statistics in your chatbot analytics, such as goal completions, it’s also important to have the user’s evaluation.
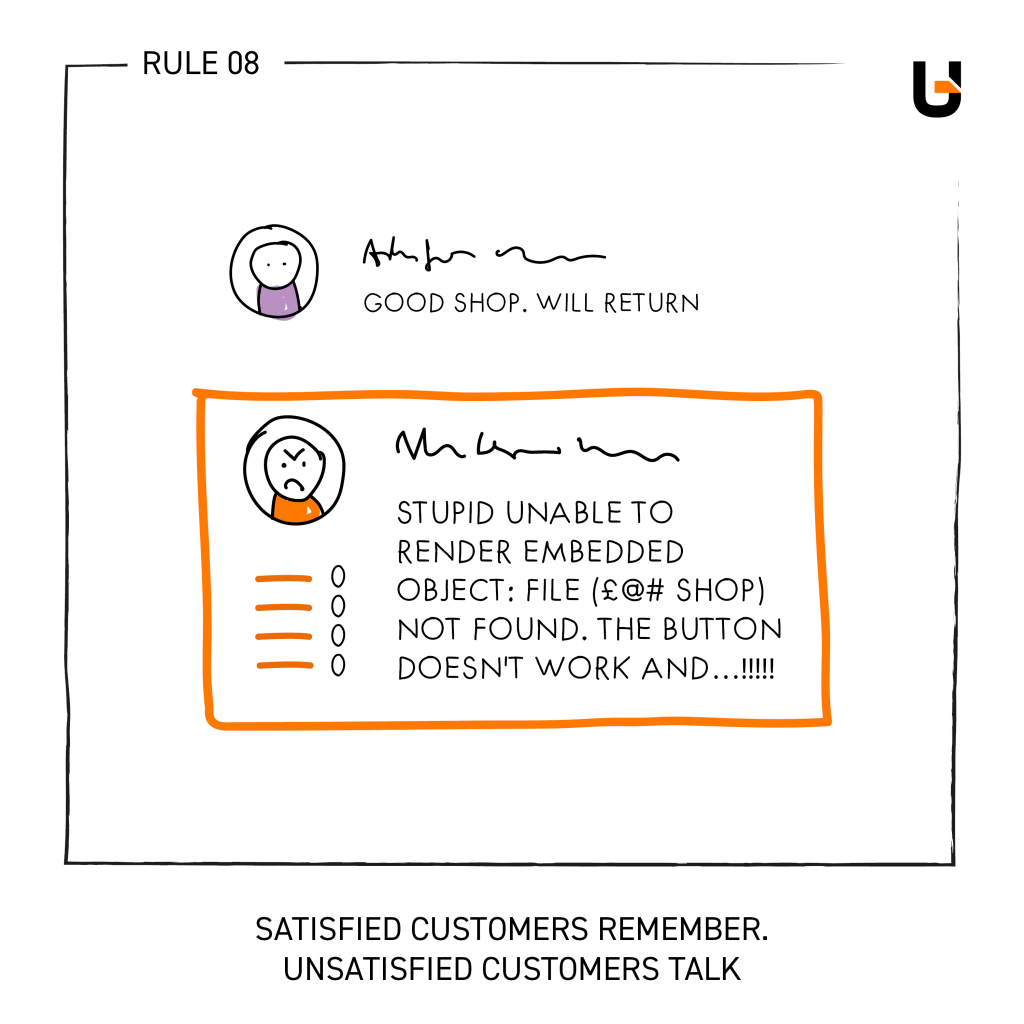
Retention Rate
We earlier mentioned the number of Engaged Users and that it’s sometimes called a Retention Rate. However, if customer retention is a critical aspect of your business, then it’s worth configuring this as a specific metric to track independently.
How to calculate it? You calculate your chatbot’s retention rate the same as any other. For a given time period, you divide the number of users at the end by the number of users you had at the start. Multiply by 100 (to express as a percentage) and you will see either a positive or negative trend. You can further customize this, for example, by focusing on specific levels of engagement.
How useful is it? If retention is vital, then this is a valuable tool. Otherwise, it’s better to focus on the Goal Completion Rate (GCR). For example, a high retention rate might not be useful for a helpdesk chatbot… as that could indicate that your customers have a lot of problems with your products or service!
Fallback Rate (FBR)
When a chatbot fails to understand a user’s input, or doesn’t know what to do next, it responds using a few default options. These range from error messages, default responses or asking the user to rephrase their query. All of these are known as fallbacks and the percentage of which can be expressed in your chatbot analytics as the Fallback Rate.
How to calculate it? Fallbacks are specifically triggered actions, so these are counted when they occur. To determine the Fallback Rate overall, however, we must compare it against the total number of interactions, not the total number of users or conversations. This is because a chatbot could fallback multiple times in a conversation.
How useful is it? The FBR is a strong indicator of your chatbot’s performance. Rather than measuring the ability to resolve goals, it shows if your chat bot is at least able to follow the conversation. A high FBR can indicate your chatbot needs improvements, or there are user needs that weren’t accounted for.
Customer Satisfaction Score
Emotional sentiment analysis isn’t the only way to determine if users are happy. When it comes to customer satisfaction, you can always ask them. Customer satisfaction scores can be fully customized – you could ask for a score out of 5, or out of 10, for example – but they follow the same principles as Net Promoter Scores for businesses.
How to calculate it? This is a custom step to should be implemented into the chatbot. You want to ask users for as simple a score as possible to ensure the most success. The more input you ask for, the less likely people will be willing to provide it.
How useful is it? Alongside user sentiment, asking for a direct score will give you immediate insight into the user experience of your bot, and how it meets user needs overall.
Human Takeover Rate (HTR)
Chatbots are not perfect. When a chatbot fails to understand the user or meet the users expectations – or the customer simply asks something unexpected – a live agent will be needed. A support agent or two should always be around during business hours (or if the above metrics show, at other peak times) to help in these situations. The Human Takeover Rate (HTR) – sometimes known as the Escalation Rate or Chat Handoff Rate – indicates how often this happens.
How to calculate it? This is another instance that will be trigged by a certain action – in this case, when chatbot users are sent to a human agent. It’s important to note that you should track when the user is sent to a support agent and not when the human agent responds. If you don’t have enough people to answer, that’s a different metric and problem.
How useful is it? Your human agents are an business resource that need to be measured. To effectively ensure every live agent is being used effectively, you need to measure how often the chatbot is passing requests through. A lower HTR score is a good indicator things are going well, and it’s a good way of improving the chatbot’s relevance and performance overall.
Commercial Metrics
Finally, it’s always worth having some metrics specifically focused on the business perspective. A chatbot, like all things, is still a business expensive that should be considered as such. Therefore, you need to not only measure your chatbot performance in terms of user feedback and satisfaction, but also a strategic investment
Leads Captured
If you want to capture leads with your chatbot, you should obviously track it as a metric. After all, you should always which channels your leads come from.
How to calculate it? You need a clear definition of a lead in your business. In most companies, especially in regards to chatbots, this happens when the user provides contact information, as this shows they are interested enough to prolong and expand contact with your business.
How useful is it? Knowing the amount of leads generated is obviously a very significant factor if that’s the purpose of your chatbot.
It’s also important to distinguish this metric from your GCR. If you set your GCR to a specific number of leads, then these metrics are showing similar results. If your capturing vastly more leads than your objective then, firstly, you can consider raising your objective. And secondly, congratulations! Because that’s an excellent ‘problem’ to have 😉
Return on Investment (ROI)
ROI needs no introduction. Calculating what you gained from an initial cost is a business fundamental. So let’s explore how to fit it into your chatbot data analytics.
How to calculate it? Here’s the tricky part. Your Return On Investment for anything is calculated the same way: you subtract the investment costs from the financial benefits. If you divide this by the investment costs and times by 100, you can express this as a percentage.

So we can determine our investment costs easily enough: the cost of chatbot development and any additional costs that you may have incurred. Expressing the financial benefits, however, is not so clear cut, as it depends on your goals. If your chatbot is designed to save your live agents time, then this needs to be expressed as cost savings. On the other hand, if it’s designed for lead generation activity, such as making sales, you need to track the sales that the chatbot specifically contributed to.
How useful is it? ROI is very useful – and very important! It’s the overall business impact of your chatbot in regards to the wider strategy and financial operations. It can also show you, over time, if the chatbot is bringing in bigger and bigger benefits. After all, after the implementation, subsequent chatbot development costs will be lower, but the gains from tweaking and improving chatbot interactions continually increase the gains.
How to Actually Track Chatbot Performance
The above metrics are a great start for your chatbot analytics, but you need to measure them on an ongoing basis. We recommend either monthly or quarterly.
It’s important to note that some of the most valuable chatbot analytics and insights are only determined when comparing these metrics in relation to each other – or other metrics from the company. For example:
- If you want to track the customer journey in regards to your chatbot, you can look at the Bounce Rate, Flow Completion Rate and the Goal Completion Rate to see how far along your audience is moving with your chatbot.
- Is your chatbot helpful? If you want to determine the success of your chatbot at meeting user expectations, you can look at the Goal Completion Rate compared against Total Users and Conversation Length. This way, you can see how many people complete the intended actions, and how long it takes to do this via chatbot interactions.
- How effective is your chatbot as a channel? You can look at your chatbot’s Total Users per each channel and compare it against views and traffic for that channel. For example, you can compare if your social media chatbot or website chatbot is used the most. In turn, this shows you where the biggest improvements may lie.
Of course, these are just a few examples and they’re incredibly broad. Just as each company is different, you need to know what you want your chatbot to achieve first. Then let this inform your chatbot success metrics.
Do you need a dedicated chatbot analytics dashboard?
As you can see, there are a lot of valuable insights to consider. Whilst a single chatbot metric or two might be easy to look at, the more you add, the harder it becomes to view all of your critical metrics and key performance indicators. At this point, customizable dashboards will become a powerful ally.
Even better, however, would be to integrate your chatbot analytics tools with other dashboards. For example, (with human agents/customer service and web stats/order fulfillment, CRM?)
The Most Important Aspect of Chatbot Analytics: Hard Data
If you track chatbot analytics, it’s important to also act on conclusions. Understanding your chatbot data is one thing, but actively seeking improvements is another.
This is perhaps more important with chatbots than other technologies. Because it learns as time goes on – both from its own results and guidance in regards to business objectives and other criteria – a chatbot’s performance changes. If you want to develop it properly, these are the key metrics to track. They’ll indicate when the chatbot is improving and, if not, where those necessary tweaks might be needed.
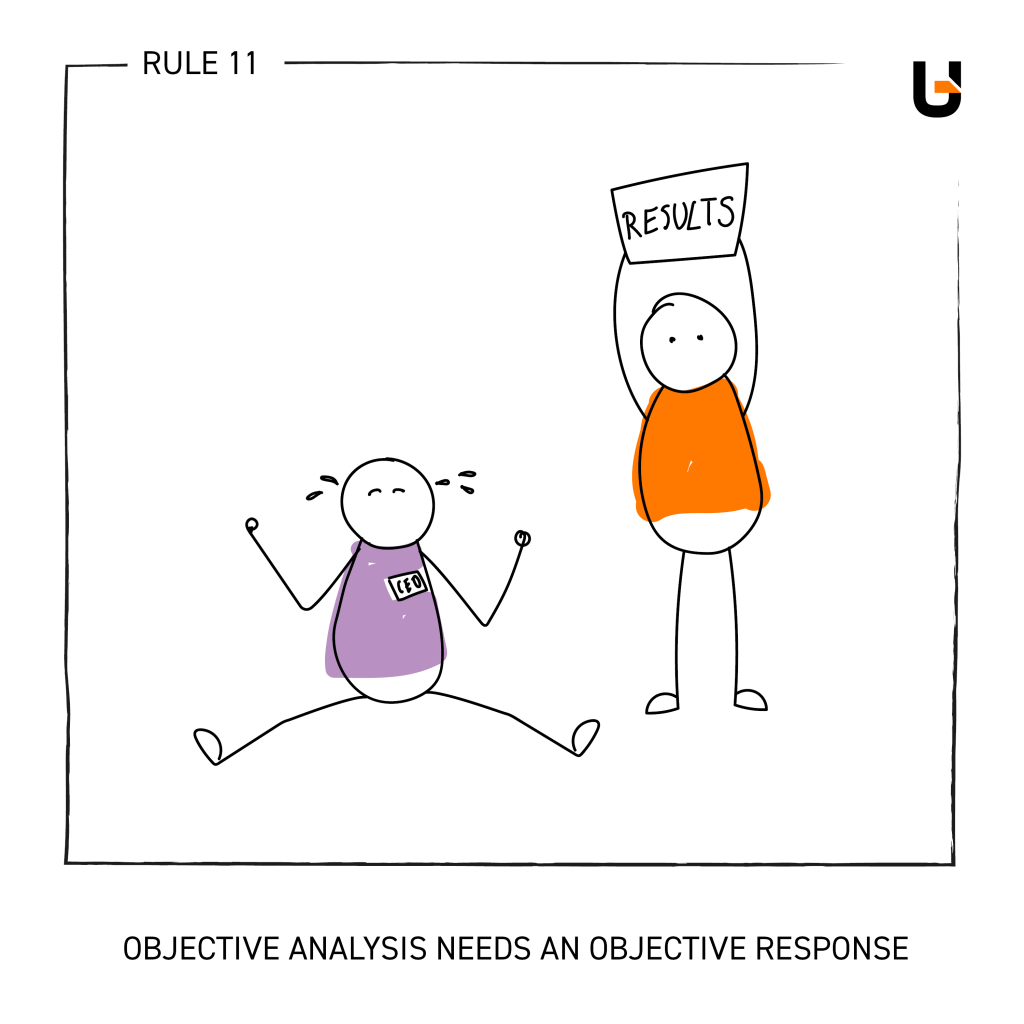
Chatbots are not magic. Even with AI, they’re still a technology. The purpose of these metrics is twofold: the first is to ensure they meet your KPIs and other objectives. The second is to help improve them to better ensure their success.